We first theoretically explore the impact of neural quantization on federated knowledge transfer across quantized DNNs and provide the convergence proof of the quantized federated learning.
F2-T DeepSLAM: Object Detection, Re-identification and Prediction with Implicit Mapping
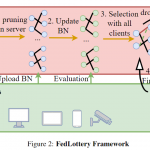