The goal of this project is to advance the point-cloud post-processing using deep learning method to understand the global and local manifolds of a 3D object.
M1-T Point Cloud Unsampling and Compression (Point Cloud Denoising)
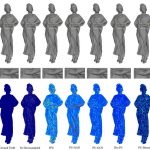
The goal of this project is to advance the point-cloud post-processing using deep learning method to understand the global and local manifolds of a 3D object.
The goal of this project is to develop novel deep learning algorithms for video segment hashing and identification to support efficient and accurate duplicates identification and removal from phones and cloud storages.
We are proposing end-to-end video compression with motion field prediction. In video-based point cloud compression (V-PCC), a dynamic point cloud is projected onto geometry and attribute videos patch by patch for compression. We propose a CNN-based occupancy map recovery method to improve the quality of the reconstructed occupancy map video. To the best of our knowledge, this is the first deep learning based accurate occupancy map work for improving V-PCC coding efficiency.
The goal of this project is to advance the point-cloud post-processing using deep learning method to understand the global and local manifolds of a 3D object.
The goal of this project is to develop novel deep learning algorithms for video segment hashing and identification to support efficient and accurate duplicates identification and removal from phones and cloud storages.
Conventional video coding methods optimize each part separately which might lead to sub-optimal solution. Motivated by the success of deep learning on computer vision tasks, we are proposing deep learning for video compression in an end-to-end manner.
We propose to tackle denoising problem using deep learning into two folds: outlier removal and denoising. We used two stage deep learning pipeline where first stage acts as a binary classifier that classifies 3d points either as outlier or non-outlier. The second stage receives non-outlier and noisy points from the first stage and learns the underlying manifold to produce residual noise from the reference(true) surface.
Advancing the state-of-the-art in image/video compression by adopting deep learning methods in prediction, transform, entropy coding and post processing. Develop fresh new coding tools based on deep learning for post processing, reconstruction enhancement. Investigate new pipelines using deep learning for end-to-end image/video compression. Achieve significant coding improvements with applicable computational complexity as well as deliver insights into deep learning video compression for machine consumption, e.g., tracking, segmentation, recognition.
Enable efficient 3D sensing and information sharing for auto-driving and smart city with 3D point cloud denoising and end-to-end compression using deep learning architectures. We would explore deep learning architecture for point cloud processing. We formulate a multi-scale CNN based 3d point cloud feature extraction technique.
This project aims to design a deep learning based end-to-end solution for dark image denoising and enhancement using image decomposition method, achieving significant improvement in output image quality. Further it aims to improve the image super-resolution by exploiting temporal redundancy. The project also aims at developing an end-to-end solution for multi-frame image super-resolution which involves image registration using optical flow and deep learning for noise removal, achieving significant gain in performance over the state-of-the-art methods.