We propose comprehensive resources and models for understanding automatically
transcribed videos. In particular, in this project, we pursue a deep learning model for identifying the
important points and questions mentioned in a video transcript. To achieve this objective, we employ two specific deep learning models.
O3-N Understand Video Transcripts in Live Streamed Videos
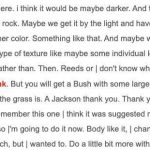