Current question answering systems is incapable of providing human-interpretable explanations or proof to support the decision. In this project, we propose general methods to answer common sense questions, offering natural language explanations or supporting facts. In particular, we propose Copy-explainer that generate natural language explanation that later help answer commonsense questions by leveraging structured and unstructured commonsense knowledge from external knowledge graph and pre-trained language models. Furthermore, we propose Encyclopedia Net, a fact-level causal knowledge graph, facilitating commonsense reasoning for question answering.
F12-A Explainable Commonsense Question Answering
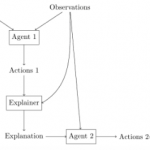