Advancing the state-of-the-art in image/video compression by adopting deep learning methods in prediction, transform, entropy coding and post processing. Develop fresh new coding tools based on deep learning for post processing, reconstruction enhancement. Investigate new pipelines using deep learning for end-to-end image/video compression. Achieve significant coding improvements with applicable computational complexity as well as deliver insights into deep learning video compression for machine consumption, e.g., tracking, segmentation, recognition.
M1-V Deep Learning in Video Compression
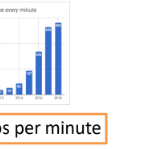