In the second year, we will focus on domain ontology-specific deep learning methods, integrating contemporary deep learning model designs with the existing SMASH healthcare ontology and corresponding data, to structure both model input and potentially output in order to develop meaningful semantic explanations that compare favorably with the state of the art.
O2-M Ontology-based Deep Learning with Explanation for Human Behavior Prediction
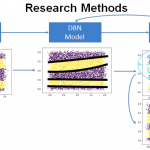