We propose a novel “Semantic Deep Learning” method to analyze the electronic health records of real patients. Our previous work as successfully used a hypergraph- based approach in the clinical text notes from Stanford Hospital’s Clinical Data Warehouse (STRIDE).
O1-N Semantic Deep Learning for Electronic Health Records
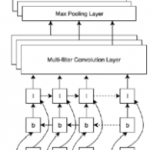