Short text Matching plays an important role in natural language processing tasks such as information retrieval (IR), question answering (QA), and dialogue system. Traditional text matching methods rely on human-crafted rules and template. Though they are effective to deal with specific situations, but lack in the ability to handle unobserved cases. In natural language, it’s infeasible to exhaust every possible expression that conveys the same ideas. Recently, neural network-based models have good generalizability to unobserved cases but provide less interpretability. In this project, we aim to balance the two sides by introducing the concept of entity to QA. We propose an interpretable, deep learning-based short text matching model for customer service domain question answering, which consists of two major components: Word segmentation enhanced Named Entity Recognition (NER), and text semantic matching. By doing so, the proposed method explicitly models entities and types of entity as building blocks of interpretable QA.
O6-N Knowledge Graph based Question Answering for Automatic Customer Service
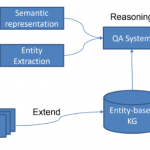