We propose a novel “Semantic Deep Learning” method to analyze the electronic health records of real patients. Our previous work as successfully used a hypergraph- based approach in the clinical text notes from Stanford Hospital’s Clinical Data Warehouse (STRIDE). Previous experiments based on ontology (i.e., domain knowledge) annotated electronic health records show that hypergraph mining is successful in finding semantic (i.e., indirect) associations. This proposed method will take the success to the next level by adding the deep learning-based embedding in place of the basic hypergraphs of the previous approaches.
O1-H Semantic Deep Learning for Electronic Health Records
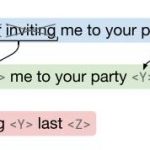